6 Preliminary Considerations for QUANTitative Social Research
Phillip Olt and Yaprak Dalat Ward
Definitions of Key Terms
- Causation: A relationship between variables, wherein one causes a change in another.
- Constructivism: The commitment that, whether objective truth exists or does not, it is only understood by humans as we construct it, which is driven by prior knowledge and social discourse.
- Correlation: A statistical relationship between variables, wherein they vary positively (when one goes up, the other also goes up; or when one goes down, the other also goes town) or negatively (when one goes up, the other instead goes down; or when one goes down, the other goes up).
- Experiment (true): A quantitative research design to test hypotheses, wherein (1) participants are assigned randomly but representatively to an experimental group and a control group, (2) all variables are tightly controlled, and (3) some treatment/intervention/experimental condition is implemented to compare data before/after.
- Hypothesis: An assumption to be tested that attempts to explain the relationship between certain variables.
- Null Hypothesis: An inverse of the hypothesis of a study wherein it is put forward that there is no relationship between the variables you are testing.
- Observational Study: A research design wherein the researchers do not manipulate or control variables; rather, data is collected based on naturalistic observations of researchers and/or participants. One example of an observational study design is the survey.
- p-value: A measure of the statistical significance of findings, wherein it is the likelihood that the null hypothesis is correct and the actual hypothesis should be rejected.
- Population: Everyone within the group being studied.
- Positivism: The belief that objective truth exists and is knowable through (and only through) scientific methods.
- Post-Positivism: An extension of Positivism, holding that objective truth exists but is only knowable by humans in part and contingently.
- Quantitative: An approach to social science research that focuses on the collection of numerical data and/or numerical analysis of data to consider relationships among variables. Often, quantitative research has the goal of producing generalizable results by performing statistical analysis of a small representative sample of the population and implying those results upon the full population.
- Quasi Experiment: Like a true experiment but without full control of the variables, which can limit the power of its findings (especially in the attempt to show cause-and-effect relationships).
- Sample: A sub-set of the population that is used to study and then generalize those results onto the population.
- Spurious Correlation: This occurs when a statistical correlation is found, but no actual correlation exists.
What is “quantitative” research?
Quantitative social research is an approach to social science that focuses on the collection of numerical data and/or numerical analysis of data to consider relationships among variables. Types of quantitative social research include experimental, quasi-experimental, and observational studies (as well as secondary analysis of data from previous such studies). Each of those types of quantitative social research will be considered in a subsequent chapter. Ultimately, quantitative social research seeks to generalize findings from a sample onto a population.
Distinguishing Quantitative from Qualitative Social Research
As the saying goes, a picture is worth a thousand words. Indeed, Zarotti (2021) perhaps most effectively illustrated the difference between quantitative and qualitative research in the Tweet pictured below. On the left side, he is dressed sharply in a suit at an academic conference with the label “Quantitative;” on the right, he is dressed extravagantly in a maroon-dyed fur coat with designer sunglasses and labeled “Qualitative.” The point being made there, somewhat cheekily, is that quantitative research has the reputation of classical academia—formalistic, clean, and respectable; meanwhile, qualitative research is the cooler and more fun uncle, perhaps looked down upon by their formalistic counterpart as not quite being good enough.
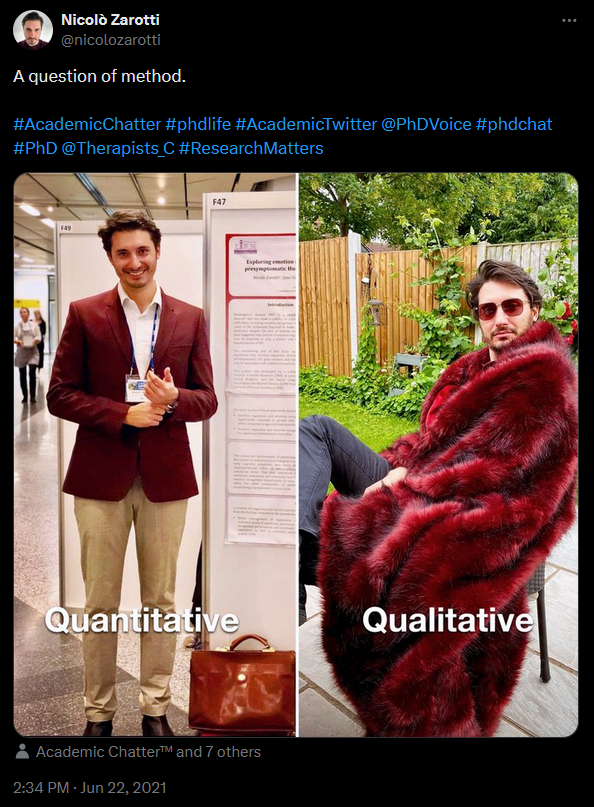
Presuppositions and Philosophical Commitments
The content of this section could—and maybe should—have been put in first chapter, as it is associated with the discussions on epistemology and ontology there. However, it is rather situated in the parallel introductory chapters to quantitative and qualitative research, as it is most applicable to understanding those methodological choices. Further, this content is severely over-simplified, and there are other approaches to these intellectual commitments that are not represented in this text; however, a certain level of detail that is both just enough and not-too-much is warranted in this level of text.
There are multiple aspects that come into play here, but there are really two and a half questions at the core:
- Does objective truth exist? If so, is it knowable?
- How do we know truth?
I often distinguish here between Truth (“big ‘T’ truth”) and truth (“little ‘t’ truth”), though that conception has evolved out of years of various readings and people. The first—Truth—considers there to be an objective truth to things, whereas the latter truth may or may not recognize the existence of an objective truth but concludes that truth is constructed by people (rather than existing independently of them). Let’s first consider the question, “If a tree falls in the forest but no one was there to hear, did it make a noise?”A Truth person would argue that, yes, a noise (i.e., sound waves) logically would have to have been made, regardless of whether we have evidence of it. A crashing tree makes noises due to the physical interactions that produce them. However, a truth person might rather argue that either we do not know if it made a noise or that it did not produce a noise if no one was there to observe it.
Waning from the philosophical, we will move on to this more practical example:
There is an accident as two cars collide at an intersection that is not monitored by video surveillance. A police officer arrives, interviewing both drivers, any passengers, and nearby witnesses. Over the course of those interviews, they get various perspectives on what happened and who was at fault.
In that example, is there an objective Truth of what happened (ex., that Driver A ran the red light, hitting Driver B’s car)? The vast majority of people would agree “yes.” Examples of alternatives to that would be (1) that none of that is real, as we live in a simulation, and (2) that one witness believes that Driver B was the one who actually ran the red light, thus having their own truth rather than an objective Truth. Then the question becomes, how would the officer find what that objective Truth is and how it came to be? Most commonly, people would answer that there will never be an absolute conclusion about what happened (for example, consider the “beyond a reasonable doubt” and “preponderance of evidence” standards in the legal system); however, it is possible to come to a sound conclusion through thorough examination of the physical evidence (i.e., natural science) and careful analysis of the various participant/witness testimonies (i.e., social science). These things then might be triangulated and weighted to come to a conclusion of variable strength.
Within this text, we will consider three approaches to truth and if/how we know it: Positivism, Post-Positivism, and Constructivism. While those three are probably the most common in social science research, that is not to suggest their superiority or that there are not others (ex., Subjectivism). Of those, Positivism and Post-Positivism are most relevant to quantitative social research, and so they will be addressed in this chapter. The qualitative chapter will include some further discussion of Post-Positivism relative to that approach, as well as Constructivism.
Positivism
O’Leary (2004) defined positivism within the research context as, “the view that all true knowledge is scientific, and can be pursued by the scientific method” (p. 10). Essentially, objective Truth does exist, and it is knowable through a rigorous application of science. Anything not known through scientific methods is not accepted as Truth (but rather as belief).
Post-Positivism
As the name suggests, post-positivists have moved past some of what construes positivism, and yet it is still a form of positivism. O’Leary (2004) noted that post-positivists “believe that the world may not be ‘knowable’. They see the world as infinitely complex and open to interpretation… science may help us to someday explain what we do not know, but there are many things that we have gotten wrong in the past and many things that we may never be able to understand in all their complexity” (p. 6). In practice then, post-positivists will generally recognize that Truth exists. However, knowledge of Truth is limited, and it is unknowable in an absolute sense. They prefer classic scientific methods (though not exclusively) to address social research questions, but they are more open to subjectivity, inductive reasoning, innovations in methodology, and multiple interpretations of reality.
Deductive Reasoning
Quantitative research generally replies upon deductive reasoning, which is often described as going from the large → small in scale. In other words, one starts from a general, accepted principle and draws a specific conclusion. For example:
- All humans need water to survive.
- Phillip is a human.
- Therefore, Phillip needs water to survive.
At some point in human history, a savvy person used this very logic to start selling bottled water. Because, if Phillip needs water to survive, he will likely want to acquire it in a safe and convenient way, which the individual-sized water bottle meets quite well. DeCarlo (n.d.) illustrated how this then translates to social science research, wherein a researcher: starts with a theory, develops an hypothesis, gathers/analyzes data, and then concludes whether or not the hypothesis is supported.
Strengths and Limitations of Quantitative Research
Strengths of Quantitative Research
Limitations of Quantitative Research
Practical Considerations
To mitigate the limitations of quantitative research, researchers must pay careful attention to research design, data collection, and analysis procedures. This includes selecting appropriate variables, sampling methods, and statistical techniques tailored to responding to the research question and objectives. Ensuring the validity and reliability of data through rigorous measurement and statistical analysis is crucial for producing credible findings. Furthermore, as in qualitative research, researchers must adhere to ethical principles (see also Chapter 2), such as obtaining informed consent, protecting participant confidentiality, and transparently reporting research findings to uphold the integrity of quantitative research.
A Healthy Dose of Skepticism
“There are three kinds of lies: lies, damned lies, and statistics.” ~Benjamin Disraeli (alleged) per Mark Twain
In this chapter on quantitative research and then the later parallel chapter on qualitative research, you will find a section encouraging you to be skeptical of published research. This skepticism should extend to multiple and mixed methods, as well as really any other research you read. Skepticism is a foundational principle of science, and so as consumers of social science research, it is essential that we read all of it as a critic and a skeptic.
Specific to quantitative research, here are some of the major concerns:
- Data integrity & authenticity
- p-hacking and manipulative analysis
- Reproducibility crisis
- Misunderstanding, misuse, and misinterpretation
- Confusing correlation and causation
- Spurious correlations
Data Integrity and Authenticity
Common across all research, there is great and growing concern about whether the data being used are real and/or accurate. There is immense pressure placed upon authors within academia to publish a lot and in top-tier journals (known as “publish or perish”). Thus, academic researchers have significant incentives to do their research quickly and come up with sensational findings that journal editors would be more likely to publish as impactful. One (unethical) way to do that is to utilize fake data. The researcher could do this manually by creating data, whether through repeatedly submitting survey forms themselves or just modifying aggregated results, that conform to accepted theories and will thus be likely published. The researcher also could use an AI bot or exploitative, underpaid labor in “click farms” to rapidly create significant amounts of data.
In the 2020s, there has been a push to increase data transparency by sharing raw data with the subsequent publication; however, that is easily subverted by actually generating the fake data. In cases where aggregated data are tinkered with, it is possible that full data sets could be analyzed by reviewers and/or readers to find those inconsistencies. But in terms of generating totally fake data, there really is no way to check.
Everything could be fake, and there is no way to know. Now, it is almost certainly not true that everything being published these days is fake, but it is certain (as evidenced by Retraction Watch, 2024: https://retractionwatch.com/) that at least some are. That said, some retractions may well be bogus themselves (ex., Ferguson, 2024).
p-hacking and Manipulative Analysis
p (or, p-value) is the statistical probability of the null hypothesis being true. So, if p = 0.049 (with 0.05 being the most common standard for significance and thus publication), then we are saying there is 95.1% confidence in there being an actual relationship between the two variables. p-hacking then is “a compound of strategies targeted at rendering non-significant hypothesis testing results significant” (Stefan & Schönbrodt, 2023, p. 1). FiveThirtyEight (n.d.) provides an excellent tool to practically understand p-hacking: https://projects.fivethirtyeight.com/p-hacking/. By selectively messing with specific variables within a large data set and then making broad inferences, one can come up with highly significant correlations quickly; however, do those correlations actually represent Truth (or even truth)? Likely not, and using the tool is somewhat of a crash course on how to lie with statistics.
Reproducibility Crisis
John Ioannidis, a medical science professor at Stanford University, is perhaps the father of the reproducibility crisis, though in the sense of uncovering it rather than causing it. Ioannidis (2005) published his bombshell paper, “Why Most Published Research Findings are False,” which became quite controversial among those who conduct human-subjects research (whether natural or social scientific research). He exposed how biases in the research community, an over-reliance of quantitative formulae, increasingly niche research and researchers, and similar factors have contributed to the increasing likelihood that most research findings are just confirming what was being sought rather than discovering what was actually True.
The concerns rapidly accelerated to a “crisis.” Brian Nosek, a psychology professor at the University of Virginia, then founded the Center for Open Science in 2013. Concerned about whether quantitative psychological studies were truly reliable as they claimed, he embarked on a journey that exposed a key flaw in quantitative social research. Unfortunately, whether a study is published in a top journal, whether the author is a world renowned expert, or most other variables, the Center for Open Science’s (2024) Reproducibility Project has yielded extremely disappointing results, specifically in psychology but now many other fields as well. Their work boils down to replicating the methods of published studies and seeing if the results are consistent with the original study’s results. While the original studies report being statistically reliable, those statistical projections of reliability have generally not held up. A great number of “reliable” findings subjected to re-testing have turned out to not be reliable. If a study is not reliable, then the findings and conclusions are of little to no use (or at least must be interpreted with the same degree of caution as qualitative studies). Given that all or almost all of our recent generalized knowledge comes from such studies, finding that many (potentially upward of 70%) are meaningless is concerning, at the least. Whether the lack of repeatable results is due to researcher misconduct (i.e., lying), poor methods, the inherent unpredictability of human behavior, or something else, we simply do not know beyond a few of those studies.
The reproducibility crisis, dating to the early-2000s, seems substantive and unlikely to go away soon, bringing into question whether we should trust quantitative social science or really generalize any specific study beyond its original context.
Misunderstanding, Misuse, and Misinterpretation
What do these numbers and symbols even mean?! Many practitioners (and researchers, if we’re being honest) crack open a new study that’s supposedly groundbreaking, only to be confronted with massive data tables that mean little-to-nothing. What good is social work research if social workers can’t read and interpret it? What good is management research if managers can’t make sense of what they’re reading? This hidden problem is that quantitative research is often so precise and technical that it becomes opaque to the end users of the findings. While there is some value to other researchers or those practitioners who can understand what they are reading, much of the value of research in a practitioner field is lost when practitioners cannot read and interpret it. Providing more interpretive instructions (ex., what each symbol means and then what that actually represents) might seem irrelevant to researchers who live in the data, but it could have great value to practitioners reading their papers—or even encourage more practitioner reading of and engagement with scholarly research in their area.
Beyond just practitioner engagement, many studies’ findings are simply misunderstood. That might mean inferring a cause-effect relationship when only a correlation has been found. That might mean exaggerating statistical findings in the media. But however and wherever it occurs, the misunderstanding, misuse, and misunderstanding of statistical data is very common and problematic.
Confusing Correlation and Causation
Correlation and causation… These two terms are dangerous when conflated, but yet they are commonly confused, even among those who know the distinct definitions.
First, let’s establish the terms’ definitions:
- Correlation: a statistical relationship between variables, wherein they vary positively (when one goes up, the other also goes up; or when one goes down, the other also goes town) or negatively (when one goes up, the other instead goes down; or when one goes down, the other goes up).
- Causation: a relationship between variables, wherein one causes a change in another.
Second, we also need to establish two important truths:
- Correlation is not equal to causation.
- Correlation does not imply causation. (Note: It is very easy to see a relationship demonstrated and infer cause-and-effect without consciously even thinking about it.)
Correlations are usually talked about as either a positive correlation (where two variables behave similarly) or a negative correlation (where two variables behave in opposite fashion). As discussed in the sub-section below on spurious correlations, a statistical relationship between variables does not always mean an actual relationship between the variables exists. Within a correlation, it is possible that Variable A causes the change in Variable B, Variable B causes the change in Variable A, or that neither variable causes the changes. Statistical correlations are relatively easy to demonstrate, and so they are very common outcomes of quantitative social science research.
However, causation (also known as, cause-and-effect) is a much higher bar for the outcome of a quantitative social science research project.
In the natural sciences, this would be accomplished through a tightly controlled setting such that only one independent variable exists at a time. For example, think of a sealed test tube filled with Liquid A that is blue. The color remains blue inside the sealed tube at room temperature for a full day. Then, it is placed over a lit Bunsen burner, which dramatically increases the temperature of Liquid A. Liquid A immediately goes from blue to clear. We then repeat this experiment identically numerous times, getting the same result. We could then conclude that the temperature increase caused the change in color.
Causation is, however, much harder to identify and prove in social research, as variables are much more difficult to control. The random assignment of participants may be very difficult or even unethical. Other variables might be introduced into the observational study, which affect the outcomes. As such, causation in social research is rarely demonstrated. While causation normally comes from true experiments, there are, however, methods for observational studies to demonstrate causation in social research. That content exceeds the scope of this text. Others have more thorough explanations, which should be consulted as further reading (exs., Mauldin, 2020; Stafford & Mears, 2015).
Spurious Correlations
One specific type of misunderstanding is interpreting an actual relationship any time two variables are statistically correlated. With most quantitative social science research being correlational in nature, it is easy for a novice researcher to assume that a statistical correlation means that there is, in fact, a relationship between two variables. However, that is misleading. A statistical correlation suggests a relationship, but it is up to the researcher’s interpretation skills to determine if such a relationship exists. For a supposedly objective process, that can be a quite subjective keystone.
Spurious correlations occur when a statistical correlation is found, but no actual correlation exists. If one compares enough data, a huge number of spurious correlations will inevitably be found. For example, consider the example below (Vigen, 2024):
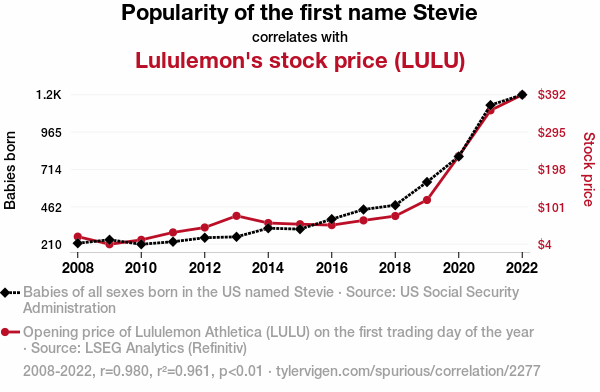
Obviously, that is a somewhat ridiculous relationship to infer. However, even when two variables are assumed to be related by a researcher and then a statistical correlation is found, a small portion will be spurious (i.e., a false positive). Given the number of published correlational studies published in a year, however, it is concerning with the number that are actually just reporting spurious correlations that were coincidentally correlated (again, see Retraction Watch, 2024).
Key Takeaways
- Quantitative research emphasizes the breadth of generalizability rather than the depth of understanding.
- Quantitative research is done through experimental, quasi-experimental, and observational studies.
- Quantitative research emphasizes adherence to natural science ideals, objectivity, rigor, and consistency.
- Neither quantitative research nor its researchers are perfect. It is the responsibility of the readers of quantitative research to carefully evaluate the research and consider its generalizability to their context.
Additional Open Resources
DeCarlo, M. (n.d.). Inductive and deductive reasoning. Open Social Work Education. https://socialsci.libretexts.org/Bookshelves/Social_Work_and_Human_Services/Scientific_Inquiry_in_Social_Work_(DeCarlo)/06%3A_Linking_Methods_with_Theory/6.03%3A_Inductive_and_deductive_reasoning
Mauldin, R. L. (2020). Causality. Foundations of social work research. Mavs Open Press. https://opentextbooks.uregina.ca/foundationsofscoialworkresearch/chapter/4-2-causality/
Chapter References
Center for Open Science. (2024). Reproducibility project: Psychology. https://osf.io/ezcuj/
DeCarlo, M. (n.d.). Inductive and deductive reasoning. Open Social Work Education. https://socialsci.libretexts.org/Bookshelves/Social_Work_and_Human_Services/Scientific_Inquiry_in_Social_Work_(DeCarlo)/06%3A_Linking_Methods_with_Theory/6.03%3A_Inductive_and_deductive_reasoning
Ferguson, C. J. (2024, September 24). Retractions have become politicized: Ideological grandstanding and corporate malfeasance threaten science. The Chronicle of Higher Education. https://www.chronicle.com/article/retractions-have-become-politicized
FiveThirtyEight. (n.d.). Hack your way to scientific glory. https://projects.fivethirtyeight.com/p-hacking/
Ioannidis, J. P. A. (2005). Why most published research findings are false. PLOS Medicine, 2(8), e124. https://doi.org/10.1371/journal.pmed.0020124
Lee, S. M. (2024, September 24). This study was hailed as a win for science reform. Now it’s being retracted. The Chronicle of Higher Education. https://www.chronicle.com/article/this-study-was-hailed-as-a-win-for-science-reform-now-its-being-retracted/
Mauldin, R. L. (2020). Causality. Foundations of social work research. Mavs Open Press. https://opentextbooks.uregina.ca/foundationsofscoialworkresearch/chapter/4-2-causality/
O’Leary, Z. (2004). The essential guide to doing research. SAGE Publications.
Retraction Watch. (2024). https://retractionwatch.com/
Stafford, M. C., & Mears, D. P. (2015). Causation, theory, and policy in the social sciences. In R. Scott & S. Kosslyn (Eds.), Emerging trends in the social and behavioral science. John Wiley & Sons. https://doi.org/10.1002/9781118900772.etrds0034
Stefan, A. M., & Schönbrodt, F. D. (2023). Big little lies: a compendium and simulation of p-hacking strategies. Royal Society Open Science, 10(2), Art. 220346. https://doi.org/10.1098/rsos.220346
Vigen, T. (2024). Spurious correlations: correlation is not causation. https://www.tylervigen.com/spurious-correlations
Zarotti, N. [@nicolozarotti]. (2021, June 22). A question of method. #AcademicChatter #phdlife #AcademicTwitter @PhDVoice #phdchat #PhD @Therapists_C #ResearchMatters [Image attached][Post]. X. https://x.com/nicolozarotti/status/1407421760249765892